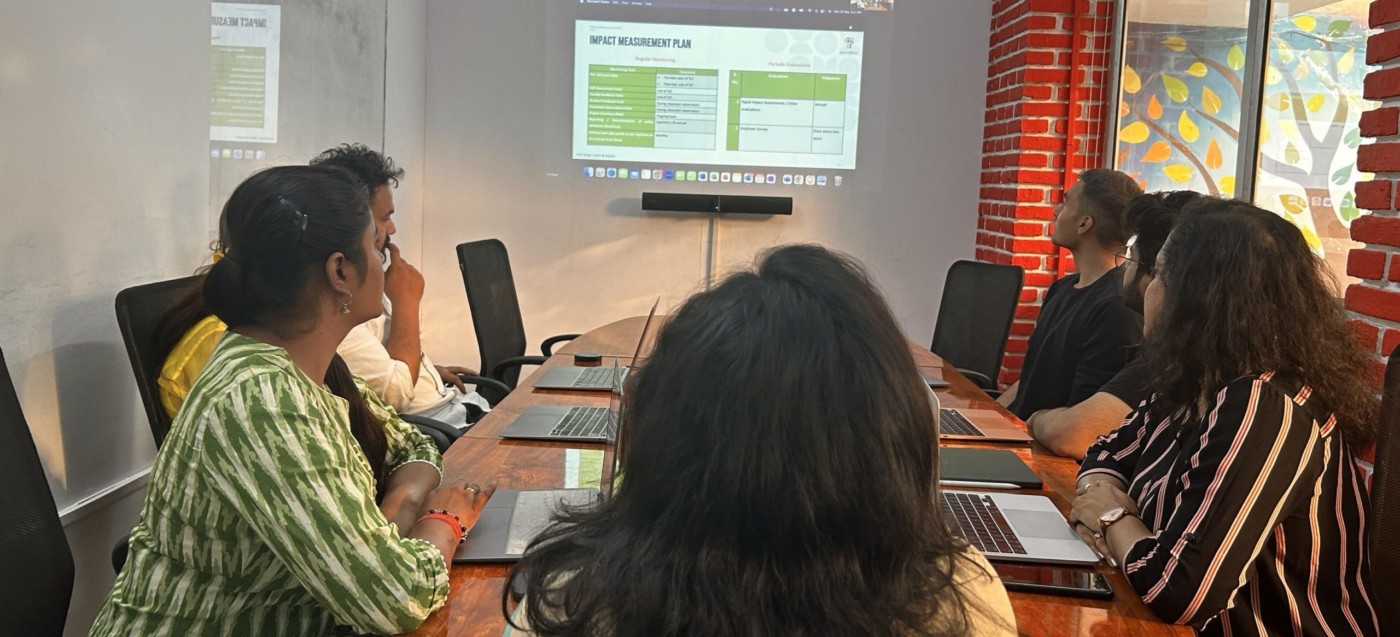
Creating a data-driven culture
This story is a part of Non Profit Work: a collection of opinions and insights to help non-profits work better and drive greater impact.
It was an enthusiastic workday in September 2020. The whole field team was to meet in person after a long time due to COVID restrictions. Usually, we would use parameters like registrations and placements to assess an area’s performance. But I wanted to try something different; go deeper.
In preparation, I had sliced data against new parameters like ‘unique students registered’ and ‘depth of relationship on campus.’ It was an eye-opening exercise for me – and as revealing for the team in our meeting. Areas that generally do well showed gaps, while underperforming areas had a depth of impact.
Every non-profit goes through hits and misses in the field. To keep motivations high, you tend to focus on the wins and ignore the losses – which is where you have insights for change. And if you’re starting out, you tend to analyze data only on a need basis: seldom treating it as an ongoing process.
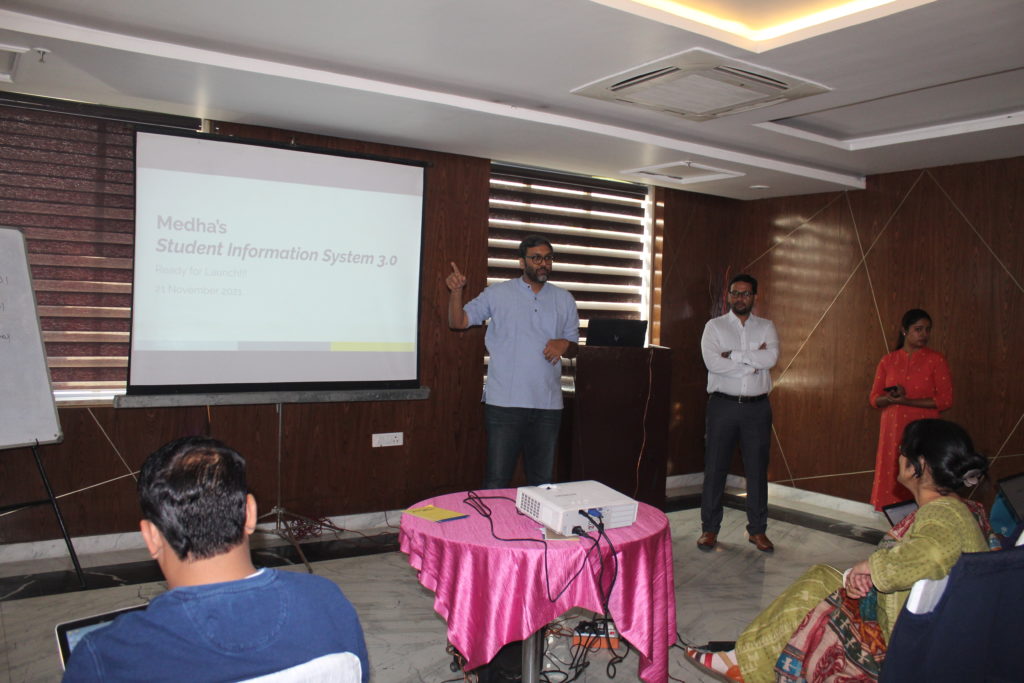
Approaching its 10th foundation year anniversary, Medha collaborated with an external agency to dig for insights from its program data. The agency started with a question for us, “Why should we believe that the quality of training and internships Medha provides is high, just because you say so? Where is the data to support this claim?”
Such questions and insights during the exercise made us realize that merely collecting program data at different levels was not enough. We relied heavily on anecdotal data and information for decision-making. “Medha was data conscious but not a data consumer,” revealed a goalkeep survey.
We were data conscious but not a data consumer.
We felt an urgent need to adopt a data culture so that we could make course corrections on an ongoing basis, update programs based on a continuous feedback mechanism, and always keep an eye on unforeseen misses.
What is data culture?
To understand what it is, we first try to understand what it is not.
Data culture is not an obsession with data. It is not collecting huge data pools to figure out what that data may be saying. Data analysis is not a niche domain anymore, nor does data culture sit within a department or a vertical responsible for organizational data.
Instead, it is about defining business problems and metrics at the design stage. In the development sector, that means defining areas of impact, indicators to reflect that impact, and tracking them for change over time. It is about asking ‘so what’ will change, ‘how’ will that change be measured, and ‘how many’ will benefit from an initiative.
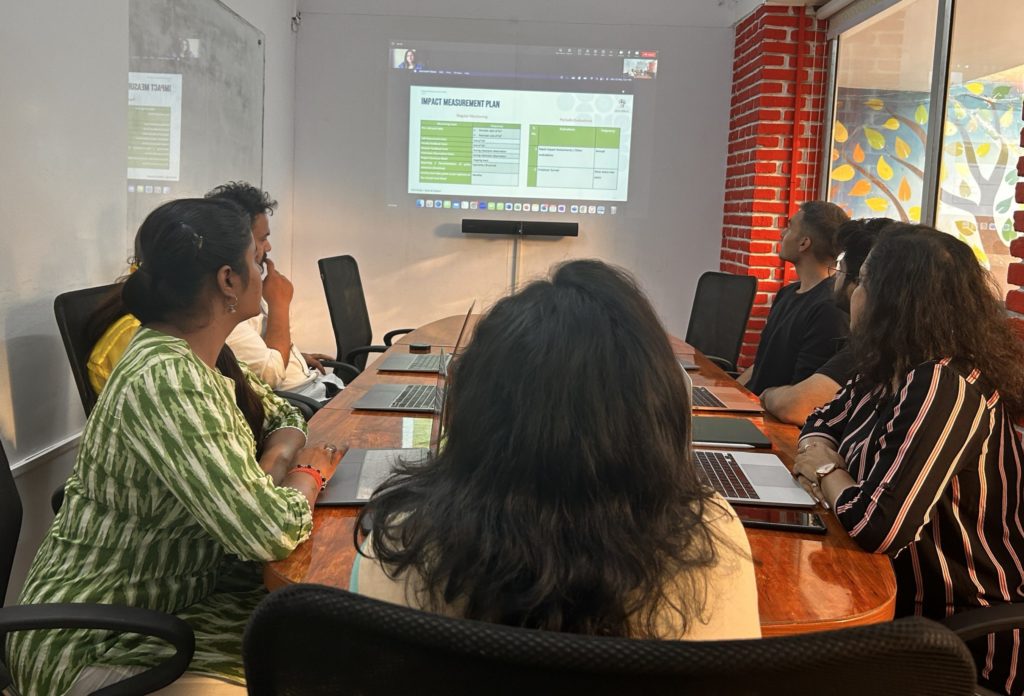
Data culture is ‘democratizing’ relevant data to foster data-based decisions at all levels of the organization. It is taking people away from feelings toward facts to form opinions based on evidence instead of instinct.
It is equipping people to tell data-backed stories, like, “58% of our internships qualify on 4 out of 5 quality parameters” instead of “We believe Medha facilitates quality internships.”
Data culture means
equipping people to tell data-backed stories.
How do we build it?
There are four pillars on which are building a strong data culture at Medha. These are strategy, technology, process, and data.
Strategy is where it all starts, where you define the objective for building a data culture, onboard people with the suitable skill set, and consistently invest in upskilling the existing team.
At Medha, our objective is to create a culture where each of us makes more informed, data-driven decisions. One way to do this is to use tools like Mulago’s Behaviour Change Map, which nudged us to think of behavioral change in terms of actions taken and be aware of our diverse motivations as a group.
Additionally, we built our Data & Impact team with people having experience in data analytics, impact measurement, and data management, along with program implementation. We housed both domain experts and adoption enablers in the team, and considered which skill sets needed to be in-house versus those that may be outsourced as required.
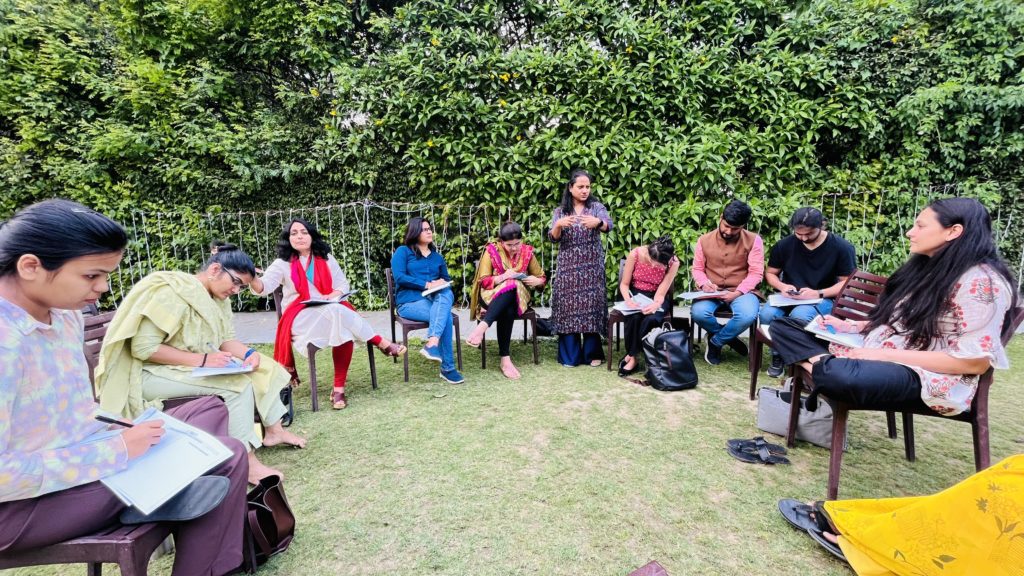
Technology is inevitable for democratizing data: making data accessible and user-friendly across the organization. It ensures that historically captured data is made available and newly captured data does not slip back into silos.
Choosing to invest in technology and yet being cost-efficient meant that we had to consider open-source solutions that are pocket-friendly, user-friendly, and effective simultaneously. So, we committed ourselves to Metabase.
While developing tech solutions, we found that a technical expert is not enough to build it alone; a “design approach” must be taken. That means working closely with the business to identify the nitty-gritty of the routines and challenges on a day-to-day basis – converting them into questions to be answered with data.
For example, while developing dashboards in consultation with the field team, our tech expert realized it is a painfully long process to identify students who need help in the program and more attention before they drop out. The team was thankfully relieved when this grueling and repetitive task was converted into a solution on Metabase, now at the click of a button.
Still, it was a two-way road of back and forth, with teams taking out dedicated time to give inputs and review solutions provided by the tech team.
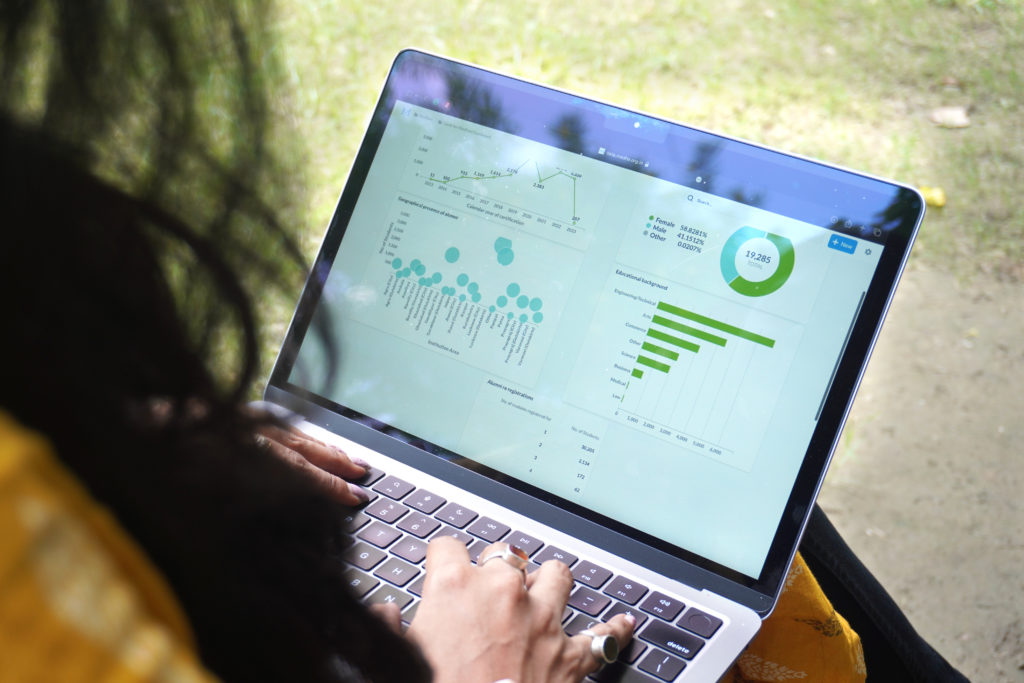
The process ensures that it is not a one-time affair but an ongoing part of your day-to-day business to collect data, analyze it, and use its insights.Though data collection becomes a mechanical process after a while, regular analysis and its consumption can be a struggle.
One solution is to establish regular points for the internal communication of insights. Share relevant insights in a manner that generates interest and builds an appetite for more. Your aim is that looking at problems and solutions from a data lens becomes a part of teams’ routines across the board.
At Medha, the process looks like monthly ‘data digests’ mailed to the entire organization, quarterly data and trends review meetings between cluster heads, and customized dashboards for different teams to track data relevant to their work.
Looking at problems and solutions from a data lens needs to become a part of teams’ routines across the board.
Finally, data is the raw material, which in today’s day and age, is available in abundance. This makes it crucial to not deep dive into all data pools but to identify what’s relevant to your current and future business problems (for example, by using log frames).
Ensure that data sets are reliable and the team trusts the data they see. Keep robust compliance mechanisms in place. Accountability for data accuracy must spread across layers – right from data capture to data approval and data management to data visualization.
Lastly, each organization has a data terminology that may be interpreted differently. So, having standard definitions of terms and maintaining transparency while communicating them is good practice – and essential for building trust.
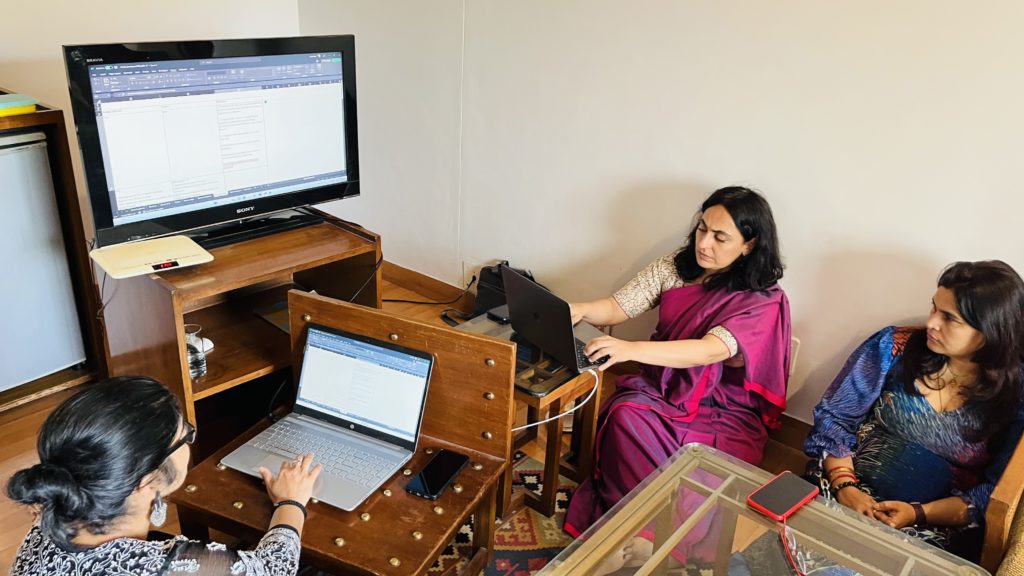
Where we are now
At Medha, we are instilling a data culture that helps us move from hindsight to insight to foresight.
Within a year of its introduction, 33% of our team accesses Metabase weekly. We are analyzing data, producing insights, and sharing it across the board. Simultaneously, more data pools are being centralized for effective analysis and visualization. We are exploring predictive models to fill efficiency gaps and considering indices to better measure our work’s impact.
It is encouraging to witness team meetings where dashboard references supplement business conversations. And it is heartwarming to see a (former tech-averse) senior employee guide his team on how to drill down into Metabase for customized information.
We have started by answering basic business questions like, ‘What happened?’ and ‘Why it happened?’ Eventually, we would be able to answer questions like ‘What will happen?’ or ‘How can we make it happen?’
That day in the conference room, everyone was reflective. There was a collective awareness that before celebrating success, let us also be aware of the granular picture or another point of view. Let’s make an impact with a deeper, uniform standard that is unquestionable and makes you conscious of where quality needs to be deepened.